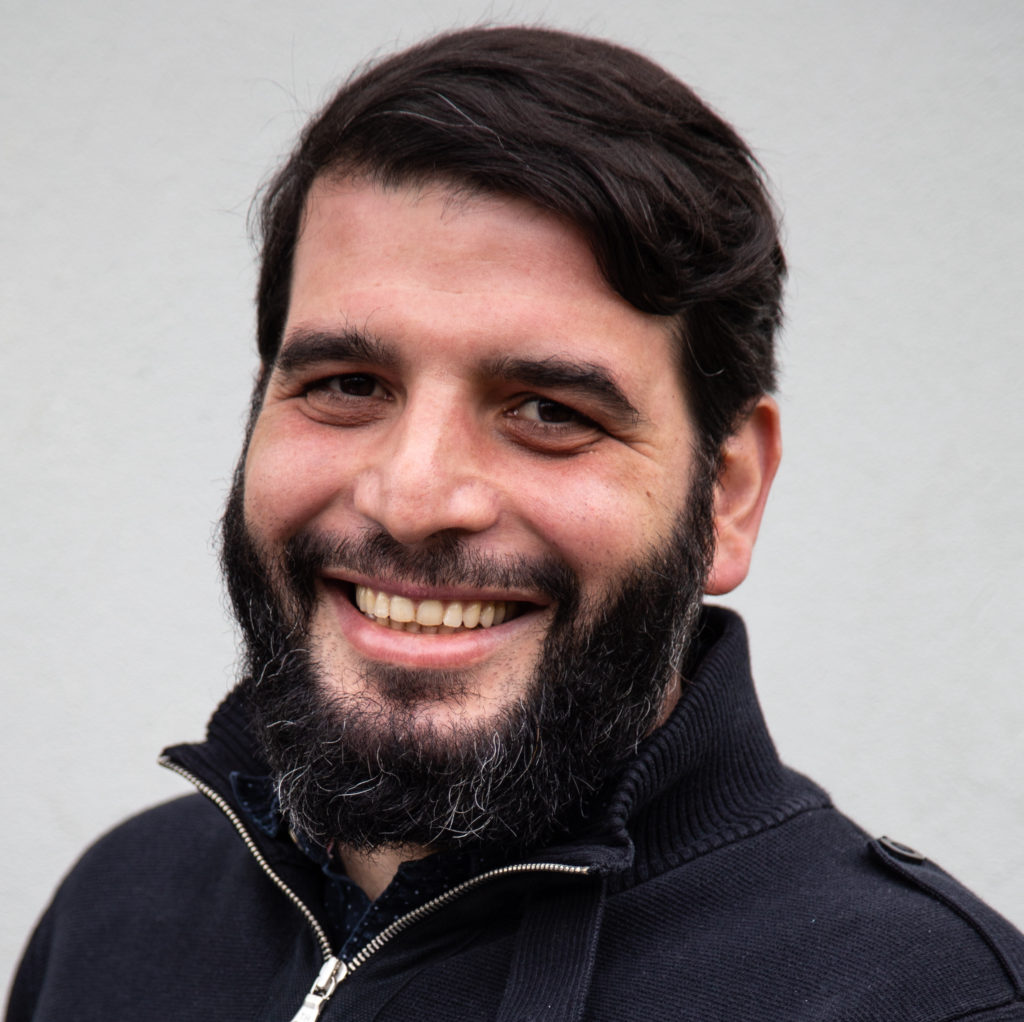
This is a summary of the novel Critical Discourse Analysis Guided Topic Modeling method published in Information, Communication & Society with application to Al-Jazeera Arabic’s coverage of the Syrian war.
A major problem in the automated analysis of topics is that context often gets side-lined. In this article, I propose a methodological intervention that I call Critical Discourse Analysis Guided Topic Modeling, bringing together critical discourse analysis (CDA) and topic modeling (TM). This method centers the often left-out context of social structures and processes. I demonstrate it examining the question of sect-based discourse. Two approaches to using this method emerge from the analysis of Al-Jazeera Arabic’s coverage of the Syrian war and its readers’ comments.
Application
My CDA-guided-TM helps identify what Brinkman calls ‘structures of discourse’ in two different but connected datasets: Al-Jazeera articles and users comments. These two applications showcase how CDA can bring context to TM analysis, which opens up the possibility for analyzing and questioning the role of structural and cultural conditions including power structures and ideology in the unfolding of events.
Applied to the dataset of 23,457 Al-Jazeera articles that explicitly concern Syria, with 125,501 corresponding users’ comments, the method captures changes in the language use by Al-Jazeera articles over time. In this first application, it opens new avenues for questions to be answered that neither TM nor CDA on their own could, such as whether there is a sect-based discourse on Aljazeera.net. It also shows that the language changes in the articles do not map on to the users’ comments. In the second application, I demonstrate that sect-based discourse is not a constant; rather, it changes with unfolding events, which contradicts mainstream literature on sectarianism. I also find that Aljazeera’s editorial shifts did not impact the sect-based narratives of its users.
Method 1: Applying TM on the full dataset
In the first method, I look at the topics extracted from the full datasets and conduct an initial analysis. To narrow that down to a more granular level, I proceed to identify topics that potentially include sect-based discourse before running TM only on the documents included in these topics for both the article and comment datasets.
Method 2: Applying TM on the dataset partitioned by year
The second method starts by assuming that the TM applied on the full datasets will generate macro-topics. To capture more specific topics, I run TM by year. For example, on the articles from the year 2010 and their corresponding comments, then 2011, until 2017, so that each year gets its own topics for the articles and the comments, and the analysis starts there.
Findings
Through these applications, I found that Al-Jazeera uses sect-based language in its content, and that articles reporting violence receive the most sect-based comments. I also found that by splitting the corpus into sub-corpora by year, not only the topics revealed are more specific, but also there is fluctuation in the sect-based discourse of the comments. This reveals the dynamic nature of what I call ‘sect habitus’, or ‘the socially constructed dispositions of sect identities that are practiced in a specific community as well as the normative ways of feeling and expressing these identities at the individual as well as the group/sect levels online and offline’.
Future extensions
While my application in this article focuses on Al-Jazeera Arabic’s coverage of the Syrian war, this method could be used to analyze any social media datasets. This could include Social Network Analysis of the top commenters to reveal their positions regarding topics of interest and extend to questions on the role of the international community, humanitarian work, ongoing events of war and violence, and peace talks, among others. There are infinite ways to use the method to answer questions about the data in these datasets, investigating the most salient topics covered, for example, by running TM on the articles that received the highest number of comments. It is also possible to measure the likes and dislikes on the comments by topic.
The significance of this method lies in its ability to both analyze large datasets of online discourse while simultaneously questioning the social structures and processes that form the contexts within which these discourses take place. Additionally, this method critically analyzes power beyond the instrumentalist approach which renders people (in this article, commenters) instruments controlled by political elites. Instead, the method enacts CDA at scale, while accounting for power relations’ dynamics in creating meaning through texts.